In the AI of the hurricane - with Dr. Chanh Kieu
Hi, folks. Welcome back to Earth on the Rocks, the show where we get to know the person behind the science. As always, I'm your host, Shelby Rader, and joining me today is Dr. Chanh Kieu. Chanh, welcome to the show.
Chanh:Yes. Thank you for having me. Of course.
Shelby:So we're gonna get to know you over drinks presumably today. And so so what would be your drink of choice?
Chanh:Yeah, I mean, coffee is the first thing that came to mind. But yeah, I was thinking about this. When I get older, I started to like a beer or something, you know, something spicy, you know. It makes sense because, you know, life is spicy, so
Shelby:That's right.
Chanh:I want to have, like, more beer now than, you know, five years ago.
Shelby:I'd say today, for folks that are listening, this this show will come out sometime in in March or early April, but we're recording on a bitterly cold day in February. So coffee feels very appropriate this morning.
Chanh:Yeah. Absolutely.
Shelby:So you're also part of this sort of diverse department that have been featured over the course of this series. And so if someone were to ask you, how do you classify yourself? How would you call yourself as a scientist? What would you say?
Chanh:Basically, I'm an atmospheric scientist, I could say. More like a theoretical and modeling side rather than experimenter side or like field experiment scientists. So I'm mostly doing research with computer programming, mathematical models, yeah, it's sort of about my research.
Shelby:So we've had some folks on the show so far who have been more on the modeling side for atmospheric sciences. Are there folks in that sort of broad atmospheric science field that are more experimentalists or field based researchers?
Chanh:Yes, we do have a number of disciplines within the atmospheric sciences that you are doing more like instrumentation, like satellite engineering and observation. And in fact, I would say a lot of people from local station, they have to do, like, measurement of, you know, like, a daily weather and report. And so they are also part of, like, observational researcher. Yeah, we do have some.
Shelby:And so the way that you describe some of the stuff that you're working on is you're, in some cases, maybe taking some of those observations that others are making, using those within some sort of mathematical model, and then using that as a predictive tool?
Chanh:Absolutely, yes. So in fact, that's the core of my research. So I basically take the observation from satellite or from local measurement, and then I try to put into the model, we call it like mathematical model, and that process they call the data assimilation. And as soon as you have observation, you put it into the model to produce the best possible initial condition you have today And then from that one, you put into the mathematical model and you try to predict weather or like hurricane intensity or track, you know, tomorrow or the day after tomorrow, like three, five days in advance. So, yeah, that part of my research.
Shelby:So, when you say you feed it into the model and try to get the best possible conditions for that day, what do you mean by that?
Chanh:Yeah, so essentially, it's the same like, if you try to predict tomorrow, you need to know what today weather is. And so the best possible way for you to do it is to collect all of the observation globally. But that step is actually very intensive because you have so much of the data and how can you possible estimate the best possible weather now to predict the future, given huge amount of information, and you need to have some sort of algorithm to do that. And data assimilation is essentially taking all of the observations globally and trying to best possible reconstruct the weather now. And before you can make a prediction for tomorrow, you need to know the weather now and start the step you have to do.
Chanh:Right.
Shelby:So this might be a question that has a difficult answer. I don't know. But when you say take all of the data observations and use some sort of algorithm to input them into the model, what number are we talking about when you're feeding these data into a model? Is it hundreds of data points, thousands?
Chanh:Yeah. I don't call number of point. I call, like, a number of gigabyte or terabyte. So it's gonna be, like, probably hundred of gigabyte every, like, few hours. And so it's the global, like, possible point you can have globally.
Chanh:And so satellite observation, for example, it covers the global. And so you have every few hours, you have like a hundred of gigabyte of data, and then you need to somehow turn that into the best possible weather now, and it's not a simple thing to do. No. And so you need to have a sort of like computer algorithm that can do it really fast because you don't want to take it like five days just to process, to guess what weather now, right? You need to do it within like five minutes or ten minutes so that you can be ready to predict weather tomorrow or the day after tomorrow.
Chanh:And so that step takes a lot of computer engineering and mathematical scheme just to do that.
Shelby:Yeah. I mean, that's impressive that you can do that at all. And and so that's you're recreating today's weather, which you can observe Yes. And then using that to predict weather
Chanh:Yes.
Shelby:For a few days out. Is that usually the time frame that you're working in? And what sort of weather phenomena are you specifically focused on?
Chanh:Yeah. My specific focus is on hurricane intensity and track and structure. So I want to see, how long in advance, how far in advance can you predict hurricane intensity. So generally for weather, if you look at the phone, you don't see they have like three weeks or four weeks weather predictions. Because simply after two weeks, then you say rain or no
Chanh:rain, it's no better than you just flip a coin. Right. So they call it limited predictability. After two weeks, you don't have any skill in prediction, but that is for general weather. So my focus is a little narrower.
Chanh:I focus on hurricane intensity, and I ask the same question, how far in advance can you predict hurricane intensity? And it may not be two weeks, in fact, it's actually about one to three days. And so if you try to predict a hurricane intensity three days in advance and you say it's Cat four, then it could be anything between Cat one to Cat five. And you cannot try I mean, at the moment, actually, cannot do better than that. So if they say Cat four, it can anything between cat three, four and five and something like that.
Chanh:And so people try to reduce that uncertainty but they can't. After three days, it's hard to do that.
Shelby:I think that, I mean, as you're describing this, I'm thinking about every time that there's a major hurricane that's gonna hit The US, which is oftentimes somewhere near The Gulf. Yeah. You see these what they call spaghetti models. Right?
Chanh:Yes.
Shelby:Yeah. And early on, the spread of those is pretty wide. But the closer it gets to landfall,
Shelby:the more constrained those get. And I think sometimes people, it feels like, get frustrated by that. But from what you're describing, that's that's just an artifact of predictability with these things. But part of what you're working on is trying to improve that or have a better handle Sure.
Chanh:I mean, it's the same thing. I mean, if I give you a coin and I say, can you just say me head or tail? Yeah, I flip it. I mean, it's suddenly you can only say with 50% head or tail because you don't have the predictability beyond, you know, like head or tail, right? And so in the atmosphere, because of the they call it the governing equation that decides the dynamics of weather and hurricane, and so they have a certain limit that we cannot predict beyond that one.
Chanh:And so like I said, example of hurricane intensity, it's simplest one, you can say cat four, but in fact it could be cat three or cat five, and after three days, then you have no ability to just say what category hurricane is going to be. Right. And so that is one part of the problem and there are many other things like precipitation, rainfall, can you just say how high the storm surge or how much rainfall you can get? Know, three days in advance, probably you can't. You can only say for twelve hours, maybe twenty four hours, yes, you can say with a good accuracy, but beyond two or three days, you just sort of like, you have to accept that you may not be able to say anything meaningful beyond, you know, a threshold.
Shelby:Yeah. So what are you and your students doing to try to sort of combat this issue?
Chanh:Yeah. So my student groups, we have, you know, I have several directions doing, but one of the directions we are using machine learning and AI to try to see how much uncertainty we can reduce using the you know, for hurricane intensity, using the data that we have. And so one example, if people predict, you know, project in the future, fifty year from now, hurricane is going to be stronger. So stronger intensity tends to be stronger, but there's a wide uncertainty when they say how much stronger it's going to be. And so we are using machine learning and deep learning to try to reduce that, so we want to say it more specifically how strong hurricanes in the future can be.
Chanh:Or if you have less number of hurricanes, how many less? We try to quantify them not just by say more or less, but by specific numbers. So that is one direction we are working on. So the other direction is as we are trying to use a model and try to improve the algorithm, I just said data assimilation. So we try to bring in all possible observation and try to see, is it true that the more observation you have, the better you predict hurricane intensity?
Chanh:And it's not always true.
Shelby:Right. I would assume there's some threshold where there's too much data. There's too many things, too many variables that you're trying to account for.
Chanh:And there's certain type of data that's more useful than the other data. So if you have satellite data, then yes, the more satellite data, the better probably you can predict the track of a hurricane. But if you talk about the intensity of the hurricane, then probably you need to have more, they call, fly data. You need to fly into the hurricane, collect more data, and then that may be useful for predicting hurricane intensity than satellite data. And so, it depends a lot on what you try to predict.
Chanh:And so, yes, it's technical but it's also practical Right. Yeah, for society.
Shelby:So you threw out a few words that I think for some people, they probably heard, but don't have a good concept of what they really So like machine learning, deep learning, and AI. Yeah. Can you just talk a little bit about sort of how you view those terms in the context of your work and how you use them?
Chanh:Yeah. That's a very good question. In fact, machine learning and AI, probably you hear that all the time now on, you know, news and media. But essentially, it's just saying that I have a bunch of the data in the past. I tried to learn the rule from the data without knowing all of the physics and, you know, mathematical.
Chanh:I just want to learn is there any rule in the data that I can learn from that huge amount of data. And so as soon as you learn the rule, you can make a prediction without even knowing all of the, you know, mathematical equation or physic equation or chemical equation. You don't need to know anything. Just learn from the rule. And this is very similar to the situation when you see grey cloud and then automatically after many years of life and you can see the darker the cloud, the more likely it's going to be heavy rain coming very soon, right?
Chanh:So you have a built in ability to predict short term weather by looking at the cloud only. Right. And so that is sort of the machine learning, you know, you just look at the data many times and then you learn from the experience that there's a rule, dark in the cloud, more heavier, then that the heavier the rainfall is going to be. So machine learning or deep learning or AI is just a different way to learn that sort of rule from the data without knowing all of the equation. And it's useful in many ways because a lot of times we don't actually know the physics or the mathematical equation of some feature, some event in the atmosphere, but we can learn from the data.
Shelby:So basically using computer assistance to look at trends in data from the past. How far back are these datasets going that you're pulling from?
Chanh:Yeah, good question. For the atmosphere science, the data that we have, that can be used probably about since 1950, probably the best one. And if you really want to use the high quality data, that can be much more useful than probably from 1980 after we have satellite data. And so they incorporate the satellite data into the atmosphere and they can reconstruct the past climate very good, back to 1980. But before that, you have data but they are not useful because they have a lot of uncertainty in the data.
Chanh:And so if you give them the wrong data for training, then it's gonna give you the wrong answer. You don't want to use that. And so a lot of times, have to use people use the data for the last forty years, you know, from 1980 up to now.
Shelby:And so whenever you're doing this sort of machine learning aspect of your work, I'm assuming I've never done this before, so these questions could be really inaccurate. You have this dataset going back to the nineteen eighties.
Chanh:Yes.
Shelby:And you have to have some way to tell a computer, I want you to shuffle through all these pieces of data and identify patterns. Are you telling the computer, look for specific patterns? Or just in general, any patterns that this computer can pick up on, notify me?
Chanh:Yeah. In fact, that's a very deep question. It depends on the scheme you are using. So in machine learning, they have two different types. One they call the unsupervised learning, the other one they call supervised learning.
Chanh:So supervised learning means you give them the pattern in the past so that it can learn. You know, it looks for the
Shelby:You identify the pattern and you'd say this is what that should look like. Find other examples of
Chanh:They're called label. So you just label all of the, you know, like the pattern in the past data and then you train the model to learn that one. The other aspect of machine learning, they call unsupervised learning, when actually you don't give any information about the label, the pattern. You just dump all of the data into the computer and let it figure that out, the pattern by itself, like a cluster of that one, and practically the more useful now is supervised learning because you just want to focus on a particular feature like hurricane intensity or you look at the atmospheric river or you look at the squalene tornado, so you have a feature you want to trace and predict. And so in that sense, supervised learning is more practical at the moment, but in the long run, I have a lot of feeling that unsupervised learning is going to take away, be as important as unsupervised learning.
Shelby:How long is this process? So you say, look through this data, either supervised, try to find hurricane intensity patterns, or unsupervised, then the computer goes off and does its thing. How long does that take?
Chanh:Yeah. It depends a lot on how much data you have and also how detailed you want to learn and depend also on the accuracy you want to say. If you just give, it's like five years of data and you just say, I just want the accuracy of yes or no, like, you know, 70%, and I just want to have a single feature or something like that. It could be really fast. It could be anything from a few seconds.
Chanh:It could be up to probably a few weeks of training. And so the training process depends a lot on what scheme, what algorithm you are using, and what feature you want, and how much data you have, and how good the label you have. If you have a random label, it will not be able to learn anything. You train it again and again after months, and it will not learn anything.
Shelby:And as you're, you know, sort of looking through and training the computer to identify these data sets, how
Shelby:difficult is it to sort of learn the language you need to talk to the computer in that way? What does that aspect of it look like?
Chanh:Yeah. At the moment, there's one programming language that is very common. Probably the most dominant one is Python. People use Python a lot for that one. And it's not hard to learn.
Chanh:In fact, they make it so easy nowadays that you can just learn the few basic things and the rest of the machine learning algorithm, if you are the user, you just want to use it, then they have like a library for you to just call and use it. Oh, that's nice. And if you want to be a developer, you want to develop a new scheme, new machine learning language, then it takes more time and you need to have a deeper knowledge about Python programming, but it depends a lot. And actually next semester, I plan to offer a class named Practice Machine Learning in Earth Science when I will show students how to put together the machine learning model the same way like you have a Lego pieces, you know? Yeah.
Chanh:So you have a different blocks built in, you know, and then all you have to do is that given this problem, I want to select these blocks and I put together to build my model. You don't have to build a block by yourself, you just use a block from a given library and then you build a model for the own, you know, application.
Shelby:Right.
Chanh:So it can be useful.
Shelby:Yeah. This is a good plug. You're if you're listening and you're an IU student, you can take doctor Kieu's class next semester. That sounds like it would be really useful. On that spectrum of, you know, being a a very basic understander of the Python language and being someone who could, you know, sort of write your own programs, where would you put yourself in terms of of how often or frequently you're using that skill?
Chanh:I would put myself in 70% for developing and 30% for using. Maybe, you know, sometimes I use more because if I use and I don't use a machine learning model and I feel like it doesn't meet my requirement, then I will spend time to develop and look deeper into that one. So it's flexible, but yeah, I would say 70% for more developing and 30% for using.
Shelby:Yeah. So you now have trained this model, you've told it in your case, identify some of these patterns related to hurricanes. Now that you've done this machine learning, deep learning to identify those trends, then what are you doing in terms of predictability after that?
Chanh:Oh, yeah. So it's more like a research question at the moment. When you do the predictability research using machine learning, then the question we try to answer is how much prediction, how much confident we can have using machine learning. Because before machine learning, you know, trend recent year, then people in weather prediction, they use a thing they call the numerical weather prediction model. And all of those models, they are very skillful already.
Chanh:And so now you introduce the machine learning, then a lot of time people question how useful the machine learning compared to the old traditional model. And at the moment, machine learning can do better than a certain aspect, but a lot of aspects, hurricane intensity for example, than traditional numerical models, are still doing better. So my job is to try to make the best yield of machine learning and try to see at what point machine learning can actually beat the traditional numerical model. And it's not an easy question because it depends a lot on how you approach the problem. And yeah, that's what I'm trying to answer.
Shelby:That's really interesting to hear because you say it in such of nonchalant way, but that has the ability to sort of change completely how weather prediction, especially hurricane prediction, may be done in the future. Do you does that sort of weight of it ever, do you ever acknowledge that?
Chanh:Yeah, I don't know. I mean, for the last two years, if you pay attention to the public and media and science publications and Google and Nvidia and all of the big tech companies, they all claim their AI model are beating all of the traditional numerical weather prediction globally. And it sounds depressing for people who do research in artificial science because they say, what else I can do? But as soon as you look into detail of the thing I call the extreme weather event, like heavy rainfall, tornadoes, squalane, hurricane intensity structure, it turns out that machine learning, the AI model at the moment, they are not comparable to the traditional model. So traditional models are still doing better than AI model, simply because the AI model, they don't have the data of those extreme events to be trained on.
Chanh:And so they realize they are based on the climate dataset that are not able to actually talk about that extreme event. And so I see a lot of opportunity. I see, you know, we can contribute and we can make a big contribution along this research.
Shelby:Yeah. Yeah. I think it's pretty an exciting field that you're in and and where you sit in that field is also sort of exciting. So some people might be wondering, you know, being in Indiana, which is a very landlocked state, how how did you get interested in in hurricanes? Why hurricanes?
Shelby:I mean, we do occasionally get the effects of the rainfall of hurricanes inland. But but, yeah, how come how come hurricanes?
Chanh:Yeah. So let me put this way. In fact, the question I I actually few people asked me the same question when I came here eight years ago already. But hurricane or, you know, heavy rainfall or any other extreme weather event, you can consider them like a symptom of the climate. So even though I'm here in Bloomington, we don't have a whole lot of hurricanes but it's the same as a doctor tries to diagnose a person, if that person is ill or like a fever, then they're going to prop some extreme, like some thing that you can see.
Chanh:Nobody can see climate change of like two degrees warmer in the future, right? But you can actually see the number of the extreme events, the number of hurricanes, how strong a hurricane is, the number of very hot days, the number of very cold days, so those extreme are the symptoms of climate change. So that's what I actually try to explain in my climate change class. I just try to tell people that you study extreme events, it actually is the same as the doctor tried to diagnose a patient's symptoms. So I'm doing hurricanes here, it doesn't mean that I have to go to the tropical region to do hurricanes.
Chanh:I can just consider that one aspect of the global climate and I can talk about the global climate, whether it's global cooling, global warming, by just looking at how strong hurricanes can be, how many of them you can see, how fast they are moving, how big they can be, how much rainfall they can dump. And so, yeah, you can study global climate by just looking at one aspect of that.
Shelby:Yeah. And is this something that you, growing up, you always thought you had an interest in sort of weather and extreme weather? Or did this happen later on? How did that come about?
Chanh:I don't know. I don't know what made me study this, but I kind of like mathematical equations that can explain things. You know, anything spin, actually I like anything spin, so I'm just curious about how to use the equation to understand anything spin. And so when I started my college As an undergrad? As an undergrad, then I was doing more like theoretical physics.
Chanh:But then I got a scholarship that's saying, Hey, you can use a computer program and then you can model the spin vortex or something like that. I was thinking that something that actually fit me and I pursued that once and then. Hurricane is natural. In other oceans, call typhoon, like in Pacific Ocean they call typhoon, and it's the same thing. Typhoon Vietnam, I'm originally from Vietnam, and we have a lot of typhoons in the past, so it also fit with the picture.
Chanh:You study something and you also see what you study can be applied for the real society and help people. So it just came to a hurricane naturally from that So
Shelby:you grew up in Vietnam and did your undergrad there. Did you ever feel the impacts of a hurricane? Did you ever sort of like lived through an intense hurricane or typhoon there?
Chanh:Yes, yes. So on and off every few years you will see a typhoon passing by my area. And so I can feel that. I mean, mostly when I was small, I just get a sense of how severe it is by looking at my parent's pipette, packing the food and locking the door and everybody was talking about that and people just tried to hide it from that one. And so that's the feeling you have about hurricanes, like something monster, you know?
Chanh:But when you study about that one, you feel it beautiful. It's the same thing with people who study virus. Everybody is scared of virus, right? But whoever does virus, they love the structure of the virus, the gene and mutation and stuff. And so, it's the same thing to me.
Chanh:Hurricane actually looked really beautiful. If you look the inside detail of it, it looked amazing.
Shelby:Yeah. I have family that lives in Florida, and they're sort of on the the West Coast of of The Panhandle. And so they very frequently will, you know, see the impacts of hurricanes, and some of them are stronger than others. They may stay, and others, they may may leave.
Chanh:Yes.
Shelby:But that's something that I've I've seen with them. One, their tolerance for those storms is so much different than mine would be. Right? Like, every time I hear about it, I'm worried about them and asking, are you all gonna, you know, head inland? And they're like, no.
Shelby:This one will be fine. We've we've had so many more that are worse. Mhmm. But also, in some ways, like, the power of those storms, even though they're really terrible and they impact a lot of people, like you say, they are there's something sort of impressive about them that this is just all happening naturally. And and what you're doing is actually looking at the reasons why, which I feel like would be, yeah, sort of an inspiring place to be in terms of research and knowing that it could eventually help folks in a lot of different areas globally in terms of predictability for things.
Chanh:Yes.
Shelby:So after you finished up your undergrad, then where did you go after that?
Chanh:Yeah. So I went to the University of Maryland in College Park for my PhD. Yeah. And then I was working as a research associate in Maryland for one year. I went to Penn State to do my postdoc.
Chanh:I went back to Vietnam to do a faculty in Vietnam. And I went back to The US and working for the NOAA, National Oceanic and Atmospheric Administration and Hurricane Group for a few years. And then I came here at IU in 2015.
Shelby:So what was that move like going from Vietnam to Maryland?
Chanh:Oh, it's a big change, yeah. When I was small, I always loved going to The US. I saw so many movies that I was thinking The US is beautiful, it's open, and I love the open and freedom of education and it's just like the science and technology part. It's just drawing me in. I love science.
Chanh:I mean, in fact, if I stay in Vietnam, my future could be very different. I could be an officer high level. But I just want to do science and I try to understand and like I said, I love using equation and to understand things and it's pretty amazing if you think about that because the earth and the atmosphere and the universe be here for five billion years, no equation is needed. But somehow, since the time we humans exist, somehow we introduce equation and we can understand it. And the crazy thing is that we can predict it in the future.
Chanh:And so when I did my PhD, I had that thinking all the time, trying to come up with some equation that can explain stuff. And I have few equations on my own that when I die, I will put it on my tomb.
Shelby:You came up with these equations on your Yeah. Yeah, that definitely should go on the tombstone.
Chanh:So, so it's just part of me loving equation and how they apply that one. And Maryland is a great place. And I've been staying in The US since 2002, so twenty years. Half of my life.
Shelby:And you also mentioned you spent some time working at NOAA, and so that's, I would imagine, a different experience than being at an academic institution. Can you talk a little bit about your time there, what that's sort of like working for a program like that and what you were doing?
Chanh:Oh yeah, that's a very good question. I was thinking a lot about that recently. I mean, yes, when I was working at NOAA, it's mostly engineering, so I just spent a lot of time fixing and improving small increments of the model aspect, try to make a better hurricane tracking intensity prediction. And they don't care about publication mostly. They don't care about, you know, teaching or anything.
Chanh:So the job is just to spend time and try to make the best model every year. And so every year, they look at how much of the track error you bring down or how much of the intensity error you can reduce. They consider as a metric of successful. At the university, you have a different task and different research. Right?
Chanh:You here, you have more freedom to research, which that's what I absolutely love. You have freedom to come up with any idea that you you can think of, and so long that it makes sense and you can bring in students and funding to support them and do teaching. And so they are very different aspects. Recently, I started to miss my time in NOAA because I feel like in that time, everything you were doing, you can see the impact next year, right?
Shelby:Yeah, almost immediately.
Chanh:Yeah. Can see how good the hurricane prediction can be, right? And so that's the feeling that I don't see it, I don't have a feeling when you are doing academic career. You are more research, it's nice and all, but on the other hand, you want to see the impact right away and it's sort of like playing a game, you know. When you see feedback right away, you have motivation to make it better.
Chanh:And that is the reason why I started to do more machine learning and AI now because that is the stuff where you can actually see impact really fast. So you do research, sometimes you can have a nice idea, you feel proud of it. I have a few that I am so proud of it. But it takes time to actually and sometimes it's a deeper understanding, it's not real application. And doing AI and machine learning can just bring back that feeling of seeing the impact right away.
Chanh:And so I have the experience on both ends, so I love that feeling, that seeing my impact right away.
Shelby:I think that that I mean, so I'm I'm a first generation college student, and I feel like that was something that that surprised me once I finally sort of got into this field at the level that I did, is that the timeline research is very long in academia. And I was just talking with someone yesterday, actually, who was visiting about a student project that they had helped lead. And for their student to go from sort of identifying this thing that they had a question about that they wanted to learn more of to then having a paper that they published was about two and a half or three years. And so it's a very long process to get that sort of feedback, like you said, to feel like you're doing something tangible, I think. So, yeah, even when I was a student, I sort of would have moments where I struggled with that, where you feel like, I'm putting in so much work and effort, but what do I actually have to show for it?
Shelby:You know, I have, like, these data that I've generated or this model that I've helped to modify, and I've started to write these things. But to actually see it through to the end takes a really long time. That's true. That's sort of hard to get comfortable with.
Chanh:Yeah. When you see impact right away, they call it feedback that can make you feel better. So that's why if you work with the outside big tech companies, they can get things done really fast compared to the university, things get slower. But outside they have feedback, they have more customers, they have an actual metric to just say, hey, you are doing good or not, right? Within six months or one year, you have to get stuff done.
Chanh:A lot of pressure, I could feel if you are working for outside company. But on the other hand, it also is driving things moving so fast. When I went to conference recently, I saw all of the best presentations or the most, like, I can see amazing presentation. It actually comes from outside companies like Google or Nvidia or Microsoft or IBM. They came and they present the results that even when I talk to my colleagues, like at university or some other government, they just say, how could they do that one within two years or one year?
Chanh:And then we could not possibly do that one at the university. They pushed the result out like that, so fast like And I'm so amazed by how fast they could get that done, the outside company.
Shelby:I would imagine that students who come through this program or other similar programs that are learning sort of these skills with machine learning or working with big data sets probably have a really good skill set to then go to some of those companies and to be employed. Whenever I was in grad school, there was someone I knew there who had done some form of climate modeling, and so they had worked with these big data sets. And it ended up after graduate school working for Google Yes. Which is not, at the time, especially, you know, like the 20, was not something that to me was very obvious. Like, oh, I have this background in climate modeling, so there's obviously a job at Google that would, you know, employ that.
Shelby:But but they were looking for people that could work with these big data sets. And so it feels like the field that you're in probably develops a lot of skills that are really useful.
Chanh:Yeah. So the student in my group, actually, they have double major and minor. They have here in Earth and Atmospheric Science department, but they also my past students, they all have data science degree, statistics, computing, mathematics minor, and some others minor. So they all have like double, actually.
Shelby:And if you had advice to give to students that are interested in this field or if you could go back to your younger self and give yourself advice, what would that be?
Chanh:Well, for the new generation of students, now they have so much more resources than my generation, so if I can come back in time, I would certainly do more math. It can help me go deeper into some of the questions that like it's mostly philosophy, you know, philosophy question, but it helped me understand. I love understanding stuff. But that is for myself, for the new generation of students, I consider they need to have more STEM and engineering education. It could be the future for them.
Chanh:And and also, even if they study, you know, art and history, if they can have some programming skill, that can be really useful for them. They can use AI to produce or help them doing some artwork or music or whatever it is. And so if you study social science or whatever it is, try to get some programming skill. It's sort of like a baby language now that we know we need to learn a second language.
Shelby:Yeah, I think that's probably been a trend on several episodes of the show is that some sort of programming knowledge is so useful across so many disciplines that whenever I was coming up through school was not commonplace to learn those things, but now feels a little more approachable for students to get that experience.
Chanh:Yes. Yeah.
Shelby:Well, that sounds like great advice across the board, which I think is a good way to bring us to our final segment of the show, which is the yes, please segment, where we each get a minute to talk about something that we're passionate about in the moment. So, Chan, do you wanna go first? Do you want me to go first?
Chanh:Yeah. So you go first.
Shelby:Okay. Yeah. I'll I'll hand over a timer.
Chanh:Yes, please.
Shelby:And if you can let me know when I have thirty seconds, fifteen, and five
Chanh:Yes.
Shelby:Then I'll try to wrap it up.
Chanh:Yep. All right. Yep.
Shelby:Yes, please. Let's get excited for outdoor yard work. I know this seems really ironic for the people in this room right now because like I said earlier, it is bitterly cold, like single digits. But by the time the show comes out, it should be late March, early April. And I love being out in the yard for the reasons that we talked about earlier.
Shelby:So much of what we do, it's hard to see immediate impacts. You know? There's no tangible evidence that you're making something or that you're collaborating and resulting in some sort of end product for a long time. But, like, actual physical yard work, you know, like mowing or weed eating or gardening, I feel the immediate impacts of that. Like, things look better, you know, the flowers eventually bloom.
Shelby:And so I just find being outdoors and working in that way so, so satisfying. And so I'm really looking forward to that aspect of the spring, which is hopefully just around the corner. Maybe it doesn't feel that way. But we're getting close, so I'm looking forward to it.
Chanh:Perfect timing. Yeah.
Shelby:Fifteen minutes. I can Yeah. I've done this, you know, once or twice before, so the timing's getting better all the time. Do you do any any outdoor yard work? Do you garden or anything?
Chanh:Yeah. I'm cleaning, you know, snow and I
Shelby:don't enjoy that nearly as much.
Chanh:Yeah. Mowing the lawn and, yeah, I do that.
Shelby:Yeah. Alright. You ready for your minute segment?
Chanh:Okay. So, I'm You
Shelby:ready? Yep. This is Chanh Kieu's. Yes, please.
Chanh:Yes, please. So at the moment, I think, student, if I want to have something to say to you, know, just go into a classroom and please don't use a phone, know, don't text and, you know, just stay focused on lecture and show respect to the peer, the friend and also learn something before it's too late because when you get older, you will look back and you'll feel you wish you could learn more. And if you don't take the time now and learn, you will regret later. Saw so many people doing, you know, when they look back, they just say, I wish I could learn more, so I want to say that one to everyone. If you can learn, please learn.
Shelby:Alright. There you go. Yeah. I feel like the the phones have become a different beast now. I can remember when I was a student.
Shelby:This is gonna age me a little bit. And we had Mhmm. No smartphones. Just, like, flip phones had just come out, which was all the rage. And so if you wanted to sneak and use your phone, you had to get very comfortable with memorizing what letters were on which number so you could, you know, under the table text your friends.
Shelby:But now every phone is like a full computer at your fingertips, so it's really easy to to have a lot of things to to look at. Yep. Maybe students will heed your call. We'll see.
Chanh:Yeah. I know.
Shelby:Well, Chanh, thank you for coming on today. This has been a lot of fun. I appreciate it. And for those listening, hopefully, we'll see you back next week. Earth on the rocks is produced by Cari Metz with artwork provided by Connor Leimgruber with technical recording managed by Kate Crum and Betsy Leija.
Shelby:Funding for this podcast was provided by the National Science Foundation grant EAR Dash2422824.
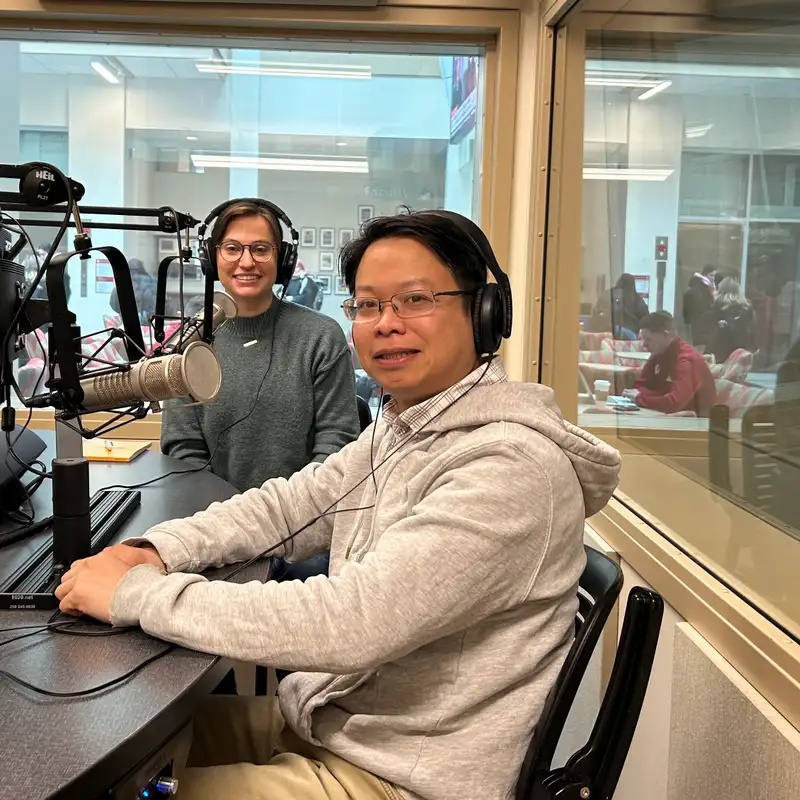